Python 打算删除大量涉及像C和C++语言那样的复杂内存管理。当对象离开范围,就会被自动垃圾收集器回收。然而,对于由 Python 开发的大型且长期运行的系统来说,内存管理是不容小觑的事情。
在这篇博客中,我将会分享关于减少 Python
内存消耗的方法和分析导致内存消耗/膨胀根源的问题。这些都是从实际操作中总结的经验,我们正在构建 Datos IO 的 RecoverX
分布式备份和恢复平台,这里主要要介绍的是在 Python(在 C++ ,Java 和 bash 中也有一些类似的组件) 中的开发。 Python 垃圾收集
Python解释器对正在使用的对象保持计数。当对象不再被引用指向的时候,垃圾收集器可以释放该对象,获取分配的内存。例如,如果你使用常规的Python(CPython, 不是JPython)时,Python的垃圾收集器将调用free()/delete() 。 实用工具
资源(resource)‘resource’ 模块用来查看项目当前得的固有的)内存消耗 [固有内存是项目实际使用的RAM] 1 2 3 | >>> import resource
>>> resource.getrusage(resource.RUSAGE_SELF).ru_maxrss
4332
|
对象(objgraph)‘objgraph’ 是一个实用模块,可以展示当前内存中存在的对象 [objgraph 文档和实例地址: https://mg.pov.lt/objgraph/] 来看看objgraph的简单用法: 1 2 3 4 5 6 7 8 9 10 11 12 13 14 15 16 17 18 19 20 21 22 23 24 25 26 27 28 29 30 31 32 33 34 35 36 37 38 39 40 41 42 43 44 45 46 47 48 | import objgraph
import random
import inspect
class Foo( object ):
def __init__( self ):
self .val = None
def __str__( self ):
return “foo – val: { 0 }”. format ( self .val)
def f():
l = []
for i in range ( 3 ):
foo = Foo()
l.append(foo)
return l
def main():
d = {}
l = f()
d[‘k’] = l
print “ list l has { 0 } objects of type Foo()”. format ( len (l))
objgraph.show_most_common_types()
objgraph.show_backrefs(random.choice(objgraph.by_type(‘Foo’)),
filename = “foo_refs.png”)
objgraph.show_refs(d, filename = ‘sample - graph.png’)
if __name__ = = “__main__”:
main()
python test1.py
list l has 10000 objects of type Foo()
dict 10423
Foo 10000 ————> Guilty as charged!
tuple 3349
wrapper_descriptor 945
function 860
builtin_function_or_method 616
method_descriptor 338
weakref 199
member_descriptor 161
getset_descriptor 107
|
注意,我们在内存中还持有10,423个‘dict’的实例对象。 可视化objgraph依赖项
Objgraph有个不错的功能,可以显示Foo()对象在内存中存在的因素,即,显示谁持有对它的引用 (在这个例子中是list ‘l’)。 在RedHat/Centos上, 你可以使用sudo yum install yum install graphviz*安装graphviz 如需查看对象字典,d,请参考: objgraph.show_refs(d, filename=’sample-graph.png’) 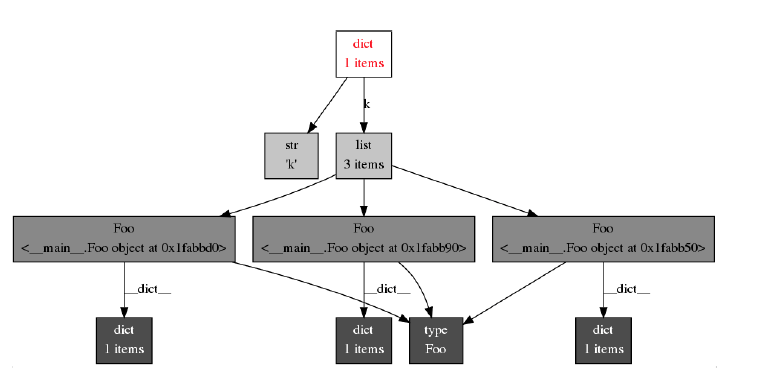
从内存使用角度来看,我们惊奇地发现——为什么对象没有释放?这是因为有人在持有对它的引用。 这个小片段展示了objgraph怎样提供相关信息: 1 2 | objgraph.show_backrefs(random.choice(objgraph.by_type(‘Foo’)),
filename = “foo_refs.png”)
|
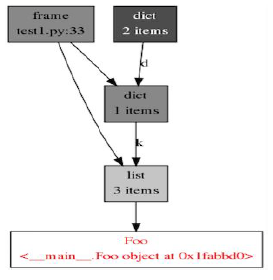
在这一案例中, 我们查看了Foo类型的随机对象。我们知道该特定对象被保存在内存中,因其引用链接在指定范围内。 有时,以上技巧能帮助我们理解,为什么当我们不再使用某对象时,Python垃圾回收器没有将垃圾回收。 难处理的是,有时候我们会发现Foo()占用了很多内存的类。这时我们可以用heapy()来回答以上问题。 Heapy
heapy 是一个实用的,用于调试内存消耗/泄漏的工具。查看 http://guppy-pe.sourceforge.net/。通常,我将objgraph和heapy搭配使用:用 heapy 查看分配对象随时间增长的差异,heapy能够显示对象持有的最大内存等;用Objgraph找backref链(例如:前4节),尝试获取它们不能被释放的原因。 Heapy的典型用法是在不同地方的代码中调用一个函数,试图为内存使用量提供大量收集线索,找到可能会引发的问题: 1 2 3 4 5 6 7 8 9 10 11 12 13 14 15 16 17 18 19 20 21 22 23 | from guppy import hpy
def dump_heap(h, i):
“””
@param h: The heap ( from hp = hpy(), h = hp.heap())
@param i: Identifier str
“””
print “Dumping stats at: { 0 }”. format (i)
print ‘Memory usage: { 0 } (MB)’. format (resource.getrusage(resource.RUSAGE_SELF).ru_maxrss / 1024 )
print “Most common types:”
objgraph.show_most_common_types()
print “heap is :”
print “{ 0 }”. format (h)
by_refs = h.byrcs
print “by references: { 0 }”. format (by_refs)
print “More stats for top element..”
print “By clodo ( class or dict owner): { 0 }”. format (by_refs[ 0 ].byclodo)
print “By size: { 0 }”. format (by_refs[ 0 ].bysize)
print “By id : { 0 }”. format (by_refs[ 0 ].byid)
|
减少内存消耗小技巧在这一部分,我会介绍一些自己发现的可减少内存消耗的小窍门. Slots当你有许多对象时候可以使用Slots。Slotting传达给Python解释器:你的对象不需要动态的字典(从上面的例子2.2中,我们看到每个Foo()对象内部包含一个字典) 用slots定义你的对象,让python解释器知道你的类属性/成员是固定的.。这样可以有效地节约内存! 参考以下代码: 1 2 3 4 5 6 7 8 9 10 11 12 13 14 15 16 17 18 19 20 21 22 23 24 25 26 27 28 29 30 31 32 33 34 35 36 37 38 39 40 41 42 | import resource
class Foo( object ):
def __init__( self , val):
self .val1 = val + 1
self .val2 = val + 2
self .val3 = val + 3
self .val4 = val + 4
self .val5 = val + 5
self .val6 = val + 6
def f(count):
l = []
for i in range (count):
foo = Foo(i)
l.append(foo)
return l
def main():
count = 10000
l = f(count)
mem = resource.getrusage(resource.RUSAGE_SELF).ru_maxrss
print “Memory usage is : { 0 } KB”. format (mem)
print “Size per foo obj: { 0 } KB”. format ( float (mem) / count)
if __name__ = = “__main__”:
main()
[vagrant@datosdev temp]$ python test2.py
Memory usage is : 16672 KB
Size per foo obj: 1.6672 KB
Now un - comment this line:
[vagrant@datosdev temp]$ python test2.py
Memory usage is : 6576 KB
Size per foo obj: 0.6576 KB
|
在这个例子中,减少了60%的内存消耗! 更多Slotting的信息,请点击链接: http://www.elfsternberg.com/2009/07/06/python-what-the-hell-is-a-slot/ |  顶 |
驻留:谨防驻留字符串!Python会记录如字符串等不可改变的值(其每个值的大小依赖于实现方法),这称为驻留。 1 2 3 4 5 6 7 | >>> t = “abcdefghijklmnopqrstuvwxyz”
>>> p = “abcdefghijklmnopqrstuvwxyz”
>>> id (t)
139863272322872
>>> id (p)
139863272322872
|
这是由python解析器完成的,这样做可以节省内存,并加快比较速度。例如,如果两个字符串拥有相同的ID或引用--他们就是全等的。 然而,如果你的程序创建了许多小的字符串,你的内存就会出现膨胀。 生成字符串时使用Format来代替“+”接下来,在构造字符串时,使用Format来代替“+”构建字符串。 亦即, 1 2 | st = “{ 0 }_{ 1 }_{ 2 }_{ 3 }”. format (a,b,c,d)
st2 = a + ‘_’ + b + ‘_’ + c + ‘_’ + d
|
在我们的系统中,当我们将某些字符串构造从“+”变为使用format时,内存会明显被节省。
关于系统级别
上面我们讨论的技巧可以帮助你找出系统内存消耗的问题。但是,随着时间的推移,python进程产生的内存消耗会持续增加。这似乎与以下问题有关: 以我的经验来看,减少python中内存消耗的比例是可行的。在Datos IO中,我曾经针对指定的内存消耗进程实现过一个工作模块。对于序列化的工作单元,我们运行了一个工作进程。当工作进程完成后, 它会被移除了——这是返回系统全部内存的唯一可以有效方法 :)。好的内存管理允许增加分配内存的大小,即允许工作进程长时间运行。
总结
我归纳了一些减少python进程消耗内存的技巧,当我们在代码中寻找内存泄漏时,一种方法是通过使用Heapy找出哪些Obj占用了较多内存,然后通过使用Objgraph找出内存被释放的原因(除非你认为他们本应该被释放)。 总的来说,我觉得在python中寻找内存问题是一种修行。随着时间的积累,对于系统中的内存膨胀和泄漏问题,你能产生一种直觉判断,并能更快地解决它们。愿你在发现问题的过程中找到乐趣! |